Improving Quality Control in the Manufacturing Industry through Digital Twins
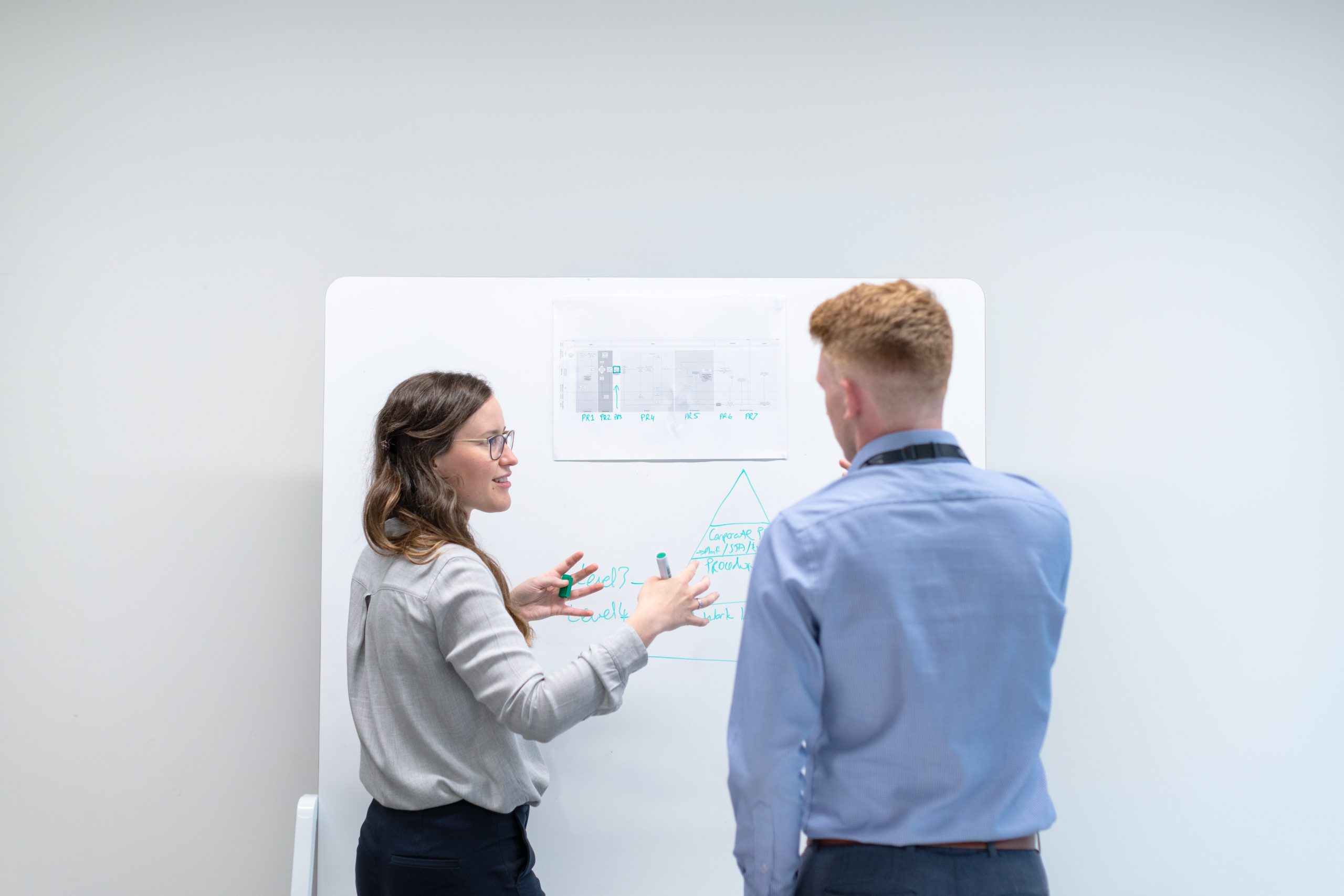
The world of manufacturing is constantly evolving, and staying up-to-date with the latest technological trends is essential. In this series, we bring you exclusive insights and expert opinions from the openZDM project partners. In this first instalment, we are delighted to showcase the University of Patras’ Laboratory for Manufacturing Systems & Automation, which also serves as openZDM’s Project Coordinator.
In the fourth Industrial Revolution, Digital Twin solutions are constantly emerging in smart factories. Although they primarily involve sensor–enabled IIoT systems that enable real-time data acquisition and through virtual replicas provide real-time monitoring, Digital Twins have great potential to improve the Quality Control process of a manufacturing business.
What is a Digital Twin?
The term Digital Twin refers to a virtual model of a physical process. A Digital Twin may use a variety of technologies to monitor physical processes. These technologies may include the use of virtual or augmented reality, 3D graphics, data modelling and sensors. Some or all these technologies, if combined, can create a replica of the physical process in the digital world.
Digital Twin implementation in a manufacturing company
Although the concept of a digital twin was introduced nearly two decades ago, at that time only companies with massive resources could take advantage of it. This has changed in the past few years and since the fourth industrial revolution many companies have implemented digital twins at various manufacturing levels, like:
- Process level: At the process level a digital twin recreates the entire manufacturing process.
- System level: At the system level a digital twin recreates, monitors and improves the entire production line.
- Asset level: At the asset level the focus is on one piece of equipment only, within the production line.
- Component level: At the component level the focus turns on a component found in a manufacturing process.
Achieving higher Quality Control through Digital Twins
Whatever the level of a digital twin implementation, they typically rely on past, present and future data. By using digital twins, company engineers can uncover potential causes for quality issues which may present during production, through the analysis of real-time data. Steps to improve quality control with digital twins may include:
- Data collection: As data collection is an essential part of a digital twin, it is a necessity to secure a fast, accurate and reliable method of collecting and storing the required data.
- Data analysis: As soon as the data collection process is established company engineers proceed to analyse the data through various data analysis techniques to be able to correlate the quality of the end product with the raw data they are provided and through potential Machine Learning algorithms predict the quality of the product.
- System modelling: In a digital twin, modelling is the core for an accurate portrayal of the physical entity. This enables the digital twin to satisfy the application requirements. This step is crucial due to the importance that a digital twin needs to be as closely depicted in the digital world as the one in the physical.
- Visualisation: To facilitate the decision-making process, engineers need to be able to visualise the effects of different inputs on the quality of the end product. This visualisation is achievable through the digital twin.
The inevitable challenges of a Digital Twin driven Quality Control
Creating a Digital Twin requires expertise and is very time and human resource consuming. This is a great challenge to overcome especially for Small and Medium Manufacturing Businesses. Moreover, the step of system modelling poses great challenges, especially in complex scenarios where a representation of manufacturing processes with dynamic physics is required.
Since an effective digital twin often requires access to big data the following challenges may also present:
- Data encryption: The data provided to the digital twin and the various Machine Learning models needs to be encrypted to assure confidentiality and prevent any possible data leak to outsiders.
- Access privileges: In case the task of creating the digital twin to facilitate quality control is assigned to a third party, the business needs to take into consideration the level of access it grants to the third party to ensure the protection of potential confidential material.
- Addressing vulnerabilities: Due to the massive amounts of data which will be used to conduct the data analysis, companies need to address any potential vulnerabilities in their systems to ensure no data is leaked.
Summarising, the quality control process of a smart factory can be improved with the use of digital twin solutions. By using digital twins and data analysis techniques engineers and factory managers are capable of analysing and visualising in real-time the effects, and possible changes of manufacturing parameters will have on the quality of the end product and achieve a lower number of defects during production.